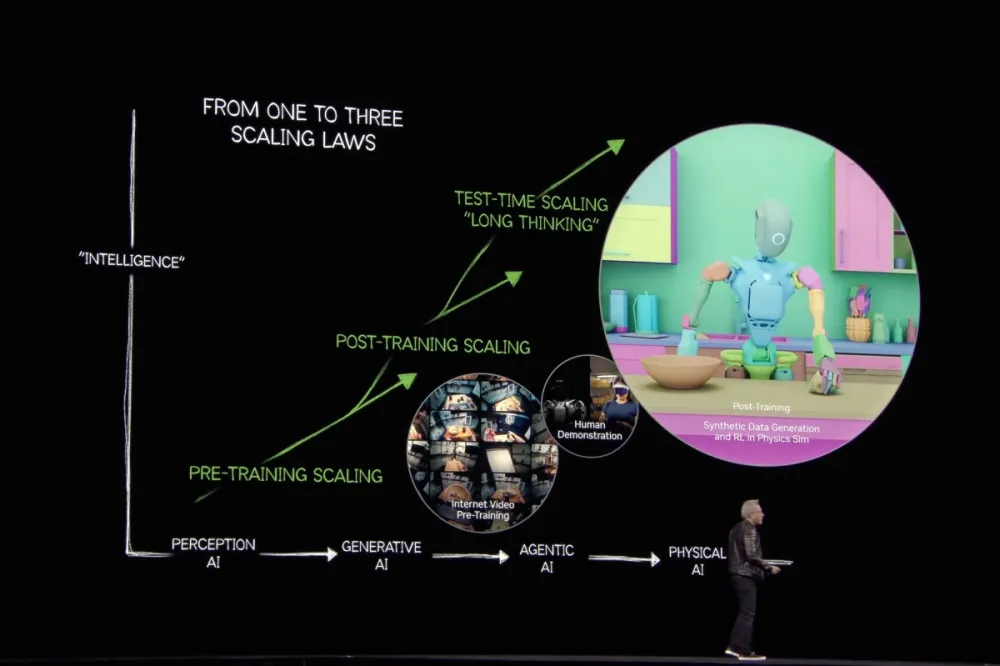
WIRED magazine, citing insider sources, has reported that NVIDIA has acquired Gretel, a synthetic data startup founded in 2019 by former members of the U.S. National Security Agency (NSA), Google, and AWS.
Neither NVIDIA nor Gretel has officially commented on the matter. However, reports suggest that the acquisition was completed for a nine-figure sum, with Gretel’s previous valuation estimated at approximately $320 million—indicating that the final purchase price likely exceeded its market valuation.
Following the acquisition, Gretel’s workforce of approximately 80 employees is expected to be integrated into NVIDIA’s ecosystem, with its technology incorporated into NVIDIA’s products and services.
Gretel specializes in synthetic data generation, a critical solution for AI developers who lack sufficient real-world data for training machine learning models. By leveraging small datasets, Gretel enables the creation of extensive, high-quality training data, addressing one of the major constraints in AI model development.
Currently, Gretel’s offerings include structured data generation, time-series data synthesis, and unstructured content creation, with an emphasis on data security and privacy protection to mitigate the risks of information leakage.
During GTC 2025, NVIDIA championed the use of synthetic data in AI training, advocating for a methodology where a minimal set of real-world data is utilized to generate large-scale synthetic datasets. This approach not only addresses the scarcity of training data but also enables the de-identification of sensitive information, reducing privacy risks.
However, opinions on synthetic data remain divided. Critics argue that biases or errors in the original dataset could be amplified when generating synthetic data, potentially exacerbating inaccuracies in AI models. Conversely, proponents believe that when combined with periodic real-world data updates, synthetic datasets can enhance training efficiency and improve overall model performance.
NVIDIA is not alone in its push for synthetic data-driven AI training. Meta, Microsoft, and Google DeepMind have also begun leveraging synthetic datasets to enhance their machine learning models.
To overcome data limitations in training robotics, NVIDIA has introduced the NVIDIA Isaac GR00T Blueprint, a system designed to generate vast quantities of synthetic motion demonstration data for robotic training. Built upon the Omniverse and Cosmos Transfer foundational models, this technology enables the creation of extensive robotic training datasets using only a small set of real-world human motion demonstrations.
According to NVIDIA, the Isaac GR00T Blueprint can generate up to 780,000 synthetic motion trajectories within just 11 hours, equivalent to 6,500 hours—or nine consecutive months—of human demonstration data.
Compared to conventional human motion data training, utilizing synthetic datasets generated by Isaac GR00T Blueprint has been shown to boost training efficiency for the Isaac GR00T N1 foundational model by approximately 40%.